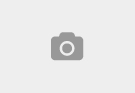
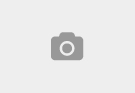
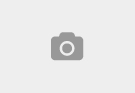
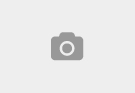
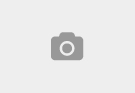
Temple Ambler’s Ultimate Esports and Gaming Hub
Level Up! Elevate your team building by visiting the campus for a day of low ropes challenge course, a mindfulness…
News
View AllTrump’s Energy Crisis: Ignoring Climate Change While Fueling Fossil Fuel Frenzy
President Donald Trump enacted numerous executive orders following his inauguration on Monday, January 20, 2025. He also proclaimed a national…
Entertainment
View All“Prepare for the Ultimate Gameplay Revolution: ‘inZOI’ Set to Dethrone The Sims!”
Referred to as “The Sims killer” by numerous online commentators and internet users, inZOI is a forthcoming life simulation video…
FUN
View AllUnforgettable Family Adventures Shine at Toronto’s Beloved Long Weekend Celebration!
– KidsFest Toronto Returns with Canada’s Largest Indoor Inflatable Festival – TORONTO, Jan. 22, 2025 /CNW/ – Dive into family…
FOOD
View AllCalgary Rises to the Top: 2025’s 5th Best Haven for Filmmakers!
Calgary is consistently reinforcing its image as a leading location for filmmakers, advancing to fifth position on MovieMaker Magazine’s 2025…