This webpage was generated programmatically. To view the article in its original context, kindly follow the link below:
http://www.the-scientist.com/artificial-intelligence-in-biology-from-artificial-neural-networks-to-alphafold-72435
and if you wish to have this article removed from our site, please get in touch with us
Previously viewed with doubt, AI earned scientists a Nobel Prize for Chemistry in 2024 after utilizing it to resolve the protein folding and design dilemma, and it has since been embraced by biologists worldwide. AI frameworks such as artificial neural networks and language models assist researchers in tackling a range of issues, from forecasting the 3D configuration of proteins to constructing novel antibiotics from the ground up. Investigators continue to refine AI models, addressing their drawbacks while showcasing extensive applications in biology.
Nobel Prize for AI: A Summary of Protein Folding and Design Journey
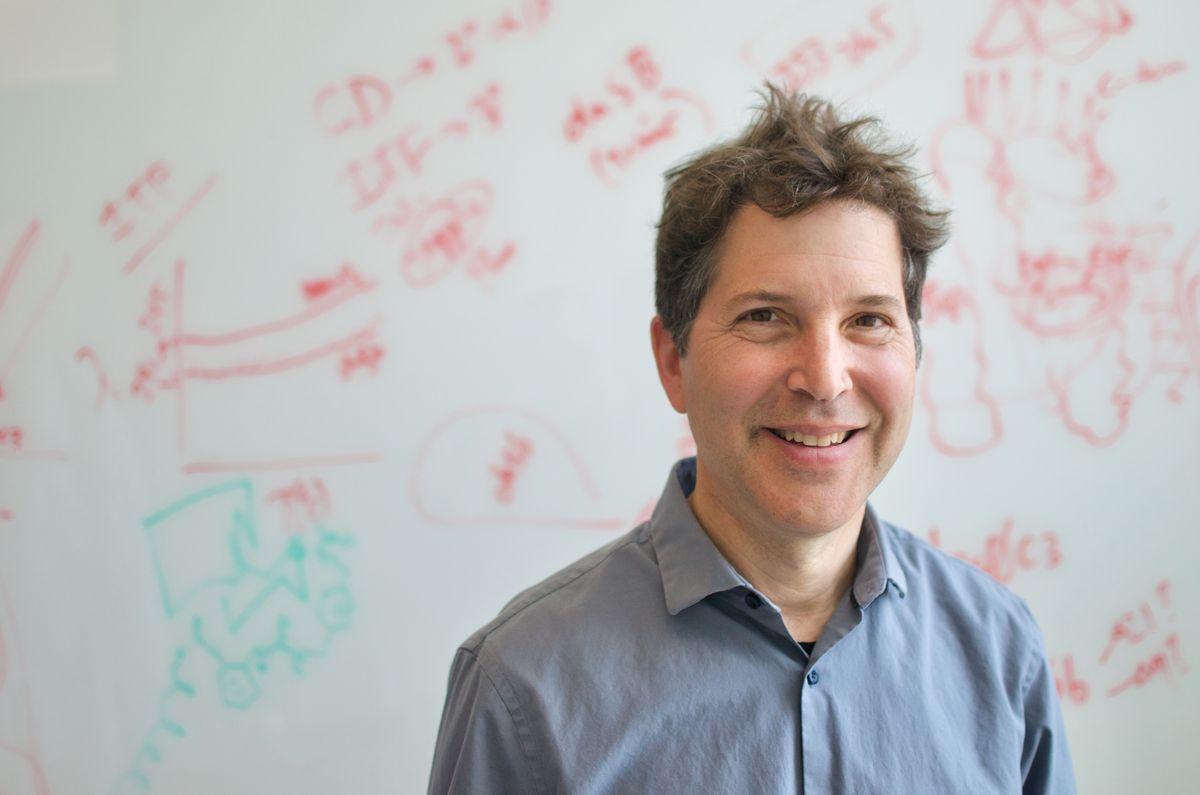
Nobel laureate David Baker employs deep learning models to develop de novo proteins that are more adept at addressing contemporary challenges than natural proteins.
Ian C Haydon
A significant point of frustration for protein researchers, the protein-folding problem has now been resolved by AI, earning University of Washington biochemist David Baker alongside DeepMind researchers Demis Hassabis and John Jumper a Nobel Prize in Chemistry. After struggling for nearly twenty years to ascertain the tertiary structure of proteins from their amino acid sequences, scientists initiated the Critical Assessment of Structural Prediction (CASP) contest in 1994 to promote collaboration in this field. In 1998, Baker’s team developed the Rosetta software for protein energy configuration modeling; indeed, a few years later, the group transformed their computational model into a game named Foldit to engage volunteers in addressing protein structures. During the 2018 CASP event, the DeepMind team introduced their groundbreaking AlphaFold program, trained using actual protein sequences and structures. Two years following, the triumph of the AlphaFold2 program in accurately forecasting protein structures led experts to proclaim the protein-folding issue largely resolved. In 2024, Baker, Hassabis, and Jumper received the Nobel Prize for their contributions that have facilitated a deeper comprehension of protein activities and applications.
Learn about the background behind the Nobel Prize-winning discovery here.
AlphaFold Accelerated the Swift Integration of AI in Biology
With the 2018 introduction of AlphaFold, an AI deep learning model, researchers were at last able to predict the 3D configuration of proteins—a long-standing hurdle in biology. Trained on 100,000 known protein sequences and structures, the model not only accurately predicts protein configurations with near-experimental accuracy but can also be utilized to design de novo proteins for various applications in therapeutics and beyond. Motivated by AlphaFold’s success, scientists are now applying deep learning models to generate spatiotemporal maps of cells, analyze cellular images to identify morphological changes indicating disease, and assess the effectiveness of new medications in ceasing disease progression to mitigate losses in the drug discovery pipeline. Experts like Maddison Masaeli, an engineering scientist and CEO at Deepcell, express enthusiasm regarding the rapid integration of AI in biology while cautioning that researchers require substantial expertise to effectively utilize AI for biological purposes.
Explore the vast applications of AI in biology here.
De Novo Proteins Address 21st Century Challenges
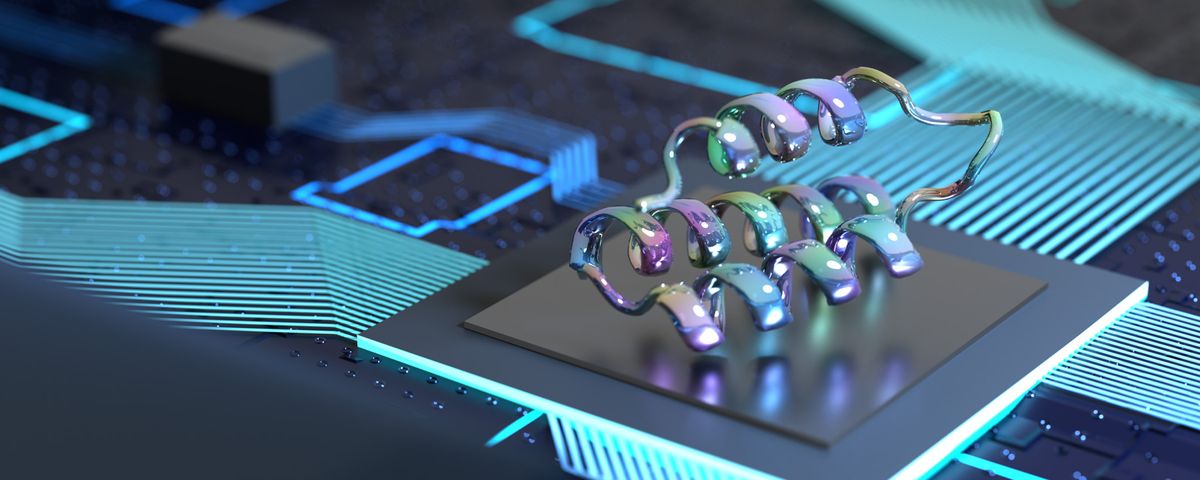
Utilizing advanced machine learning tools, researchers can formulate artificial proteins with novel functions.
Ian C Haydon
Leveraging the capabilities of AI models, scientists can now design custom proteins with distinct biological functions, enabling them to tackle problems that existing natural proteins cannot solve. Conventional protein engineering relies on making gradual modifications and assessing their impacts, while machine learning models can both create superior proteins and significantly accelerate the process. Protein design expert David Baker and his team at the University of Washington employed various AI frameworks to create stable luciferase enzymes capable of binding with synthetic luciferin for luminescence, applicable in the deep imaging of animal tissues. Although this method of protein design still holds room for enhancement and is not yet fully automated, it may pave the way for the future creation of diverse proteins for therapeutic and other uses.
Discover more about de novo proteins here.
AI Uncovers a New Antibiotic for Drug-Resistant Bacteria
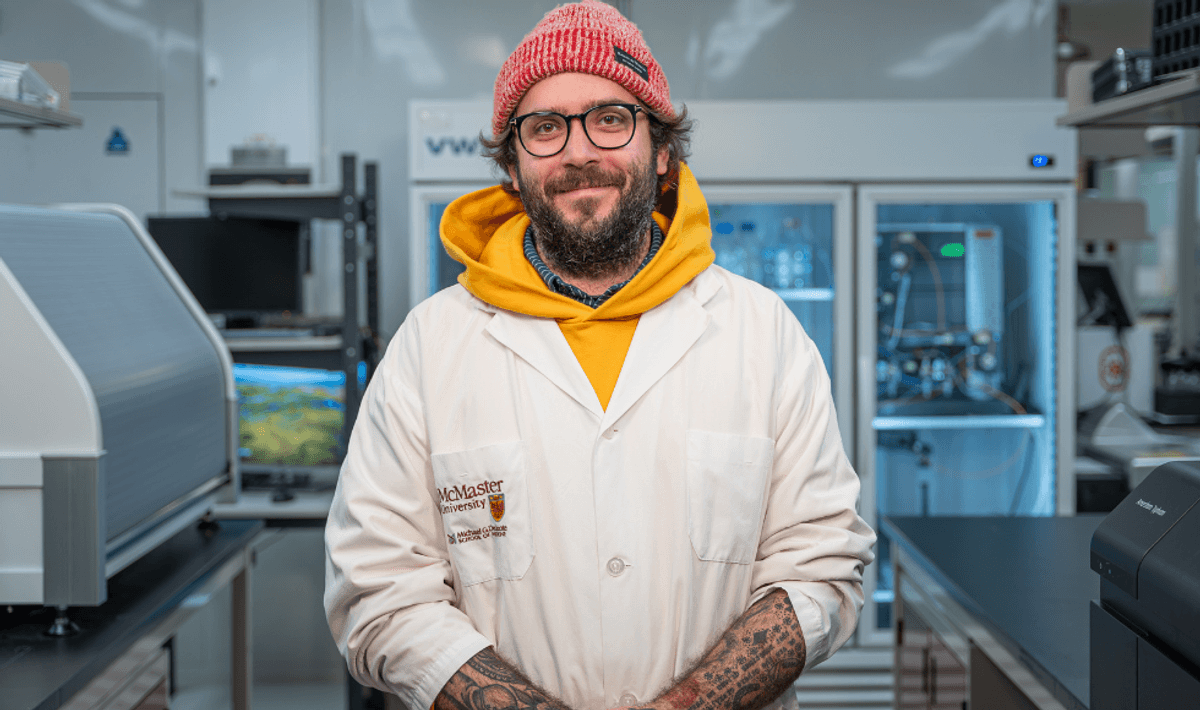
Jon Stokes and his team created SyntheMol, a generative artificial intelligence model they employed to devise novel antibiotics with anticipated effectiveness against the ESKAPE pathogen, Acinetobacter baumannii.
McMaster University
The creation of de novo proteins through AI could significantly aid antibiotic development. With the rise of antimicrobial resistance globally and a shortage of newly discovered antibiotics, researchers at McMaster University have turned to AI for designing novel antibiotics that can be readily synthesized. Headed by biochemist Jon Stokes, the team devised a generative AI model named SyntheMol to formulate small molecules that exhibit antibacterial properties against Acinetobacter baumannii, a drug-resistant pathogen recognized by the World Health Organization as a significant threat to global health. While these molecules have yet to undergo testing in human subjects, several demonstrated the ability to inhibit the growth of the targeted bacteria, as well as other drug-resistant microorganisms, in vitro.
Investigate AI-generated antibiotics in this article.
Artificial Neural Networks Mimic Human Brain Learnings
Inspired by human cognition, artificial neural networks (ANNs) represent a category of machine learning model composed of multiple layers of interconnected nodes (or neurons) capable of processing data. Each node within the network conducts a mathematical computation using weighted input data and decides whether the output will be forwarded to the subsequent layer of nodes based on a predetermined threshold value. Researchers train the ANN using datasets with known values or characteristics, then permit it to evaluate its predicted outputs againstthe accurate response for each instance so it can enhance its precision over time. The ANN can subsequently be utilized to forecast results from fresh datasets. Notwithstanding some significant constraints, ANNs can recognize patterns in intricate data that individuals may not be able to discern and carry out repetitive tasks to liberate time for researchers.
Discover more about neural networks in this explanatory article.
Large Language Models Aid in Understanding the Brain
Scientists have recently devised a language model—the kind of deep learning model integral to ChatGPT—that can infer an individual’s thoughts from MRI scans of their brain. Alexander Huth, a researcher at the University of Texas at Austin, formulated the method with the aim of enabling individuals who cannot speak to convey their thoughts, but it has also uncovered revelations about the workings of the human brain. Huth’s model demonstrated that all regions of the brain leverage meaning-related information even if MRI images indicate that solely the prefrontal cortex is engaged. While the model isn’t applicable across various individuals, implying it can’t decipher minds, authorities urge prudence as these models advance in accuracy in the future.
Explore more about language models and their relevance in biology in this article.
Forecasting Gene Expression with Artificial Intelligence
While ChatGPT is employed to anticipate the subsequent words in a phrase, scientists have developed analogous deep learning models that can predict gene expression in individual cells. Conceived by computational biologist Bo Wang and his team at the University of Toronto, the single-cell generative pretrained transformer (scGPT) is capable of examining single-cell RNA sequencing data more adeptly than many of the most widely used current techniques. The model was also more precise in predicting the impacts of genetic alteration than a conventional model. Initially trained on bone marrow and immune cells, a new version of scGPT has now been customized for the evaluation of a range of other cell types and could be employed to resolve crucial biological inquiries in the foreseeable future.
Keep reading about scGPT here.
AI models hold immense promise in biology, from assisting us in deciphering the brain to developing innovative therapeutics; nevertheless, specialists have cautioned that their application should be approached with care, and their efficacy hinges on having extensive knowledge. Researchers persist in exploring, advancing, and fine-tuning deep learning models for diverse uses, including the analysis and forecasting of biological data.
This page was generated programmatically; to view the article in its original form, please visit the link below:
http://www.the-scientist.com/artificial-intelligence-in-biology-from-artificial-neural-networks-to-alphafold-72435
and if you wish to have this article removed from our site, kindly get in touch with us